Explainable AI, Explained
Artificial intelligence (AI) is quickly becoming an essential part of many industries. However, as AI systems become more complex and sophisticated, they become increasingly difficult to understand and interpret. As such, there is a growing demand to develop AI models that are more transparent and interpretable, so that people can understand the rationale behind their decisions and trust their accuracy and fairness. This is where Explainable AI (XAI) comes in.
What is Explainable AI (XAI)?
Explainable Artificial Intelligence (XAI) refers to a set of techniques that make the decision-making process of AI systems more transparent and understandable to people using them. While traditional AI models focus on optimizing performance without necessarily explaining how decisions are made, XAI sets out to provide a clear and interpretable rationale for the decisions made by AI models.
Black-Box AI
On the other end of the spectrum, black-box AI refers to machine learning models whose decision-making processes are opaque or inaccessible, hence the term “black box.” In a black-box AI system, the internal workings of the algorithm are invisible to the user, making it difficult to understand how a model makes its decisions. This lack of transparency can lead to several challenges, including making it difficult to troubleshoot, identify bias, and take accountability.
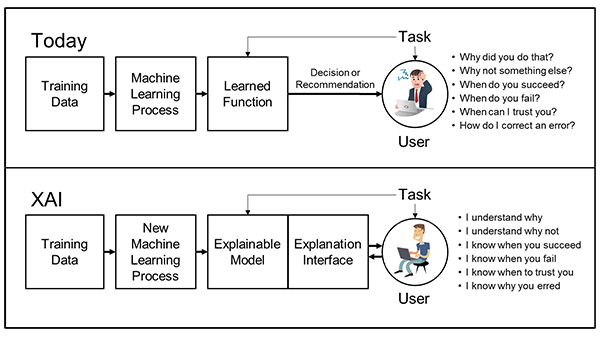
Why Does XAI Matter to Public Agencies?
AI has the potential to improve the way government agencies operate and provide services to citizens. By automating routine tasks and providing insights to policy-makers, AI can increase efficiency and aid government decision-making. However, the implementation of AI in the public sector, where transparency and accountability of decision-making are essential, it also comes with concerns and risks.
As we touched on above, traditional AI systems can perpetuate bias and discrimination depending on how they were trained and designed. Moreover, the inability to hold AI accountable for its decisions can lead to a lack of trust in the technology and in the agencies that use it. AI explainability and/or auditability can help governments combat these risks and build public trust and accountability, helping to promote the responsible adoption of AI in the long term.
Best Practices
In their 2020 State of AI report, McKinsey asserts that “companies seeing the highest bottom-line impact from AI exhibit overall organizational strength and engage in a clear set of core best practices.” Public agencies are no exception. In the public sector, some best practices might include:
- Starting simple. Identify areas where AI can be applied to improve operations, for example, chatbots for customer service.
- Developing a strategy. Outline the specific objectives you want to achieve through AI.
- Establishing ethical guidelines. Include guidelines around transparency, fairness, accountability, and privacy and hold any vendors you work with accountable to these guidelines.
- Being aware and understanding the laws and policies around AI in your region.
- Testing and monitoring AI systems regularly to ensure they are working as intended. This can include regular audits, testing for bias, and continuous monitoring of system performance.
Like any new process or technology, it is important for government agencies to carefully consider the benefits, challenges, and their own internal governance policies in place around AI before implementing it in their operations.
How UrbanLogiq Helps
At UrbanLogiq, we understand the importance of transparency and audibility in AI solutions. We believe that our customers should clearly understand how our AI algorithms work, how they make decisions, and what data the findings are based on. This is why we prioritize building AI models that are not only accurate but also auditable so that our public sector partners can responsibly capitalize on the benefits of AI without sacrificing accountability or trust.
Looking Ahead
In conclusion, Explainable AI (XAI) is a vital aspect of the growing field of artificial intelligence. It allows us to understand and interpret the decisions made by AI algorithms, providing transparency and accountability. XAI is increasingly important for applications where decisions can have significant impacts on individuals and society as a whole, such as healthcare, law enforcement, or in the public sector.
By enabling users to understand how AI makes decisions, we can identify and correct errors, and better ensure responsibility and fairness.