AI in the Public Sector: Exploring Key Subfields and Applications
The buzz around Artificial Intelligence is undeniable and has caught the attention of governments around the world. Public sector leaders recognize its potential to revolutionize decision-making. However, adopting AI in the public sector involves navigating complex ethical, transparency, and accountability issues. In this blog, we explain the different subsets of AI and their application in government contexts. We explore how government agencies can responsibly harness AI’s power to enhance public service while being aware of its challenges and limitations.
Under the Umbrella of Artificial Intelligence
Artificial Intelligence (AI), often used broadly, encompasses a variety of subsets, each distinct in its capabilities and specialized applications. Below, we break down machine learning, neural networks, deep learning, generative AI, computer vision, natural language processing, and fuzzy logic. We aim to provide a clearer understanding of their unique roles and impacts.
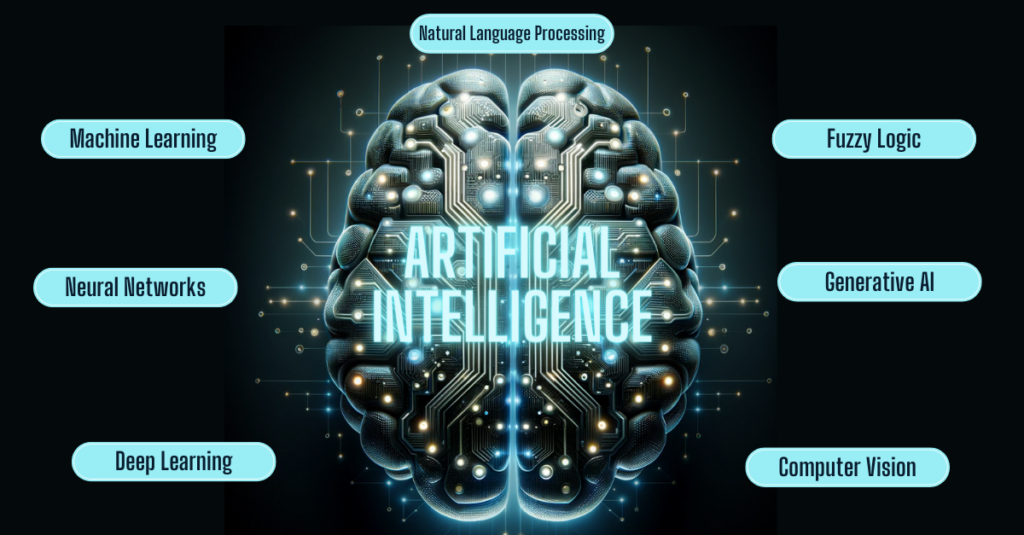
Image Source: DALL-E
Machine Learning
Machine learning is a subset of AI where computers learn to do tasks by themselves without being directly told how. It works by using algorithms and models to spot patterns in data. First, the computer is trained using a set of data, then it uses what it learned to make decisions or predictions. This method gets better over time as the computer sees more data and learns with new data. Machine Learning is especially good for tasks with smaller amounts of data that need careful analysis. It’s a great tool for governments to use when they have limited but detailed data and need insightful outcomes. For example, machine learning can be applied to historical traffic data to predict future traffic volumes.
Neural Network
Neural network refers to a computer system modeled after the human brain, used in Artificial Intelligence. It’s made up of layers of nodes (like neurons) connected in a network. These nodes process data by passing signals to each other, similar to how neurons in the brain communicate. The network adjusts its structure based on the data it processes, allowing it to learn and make decisions. Neural networks are the foundation of deep learning models and are especially effective at handling complex patterns in large datasets. They are key in tasks like image recognition and language processing.
Deep Learning
Deep Learning, while powerful in pattern recognition, often acts as a ‘black box,’ making it challenging to trace its decision-making. It’s on the other end of the spectrum and thrives on vast data sets using structures called neural networks, inspired by the human brain, to process data in complex ways. These networks go through many layers of processing to make sense of large amounts of data helping the system learn and recognize patterns to make decisions from massive datasets.
It’s particularly good at tasks like image and speech recognition, where it can identify patterns too complex for simpler algorithms. Deep Learning models act like a “black box” so it may not be practical, especially in situations where transparency and understanding the decision-making process are important. But when the impact of the decisions is considered ‘low risk’ deep learning can be quite useful. For example computer vision cameras using deep learning to identify and classify vehicles.
Generative AI
Generative AI is a type of Artificial Intelligence that creates new content, whether it’s text, images, or audio. It takes what it has learned from existing data and uses it to generate new, original outputs.
It works by learning patterns and features from a large set of data and then using that understanding to create new data that resembles the original set. Generative AI is used in a variety of applications, like creating realistic computer graphics, new music, simulating human speech, or writing text. ChatGPT uses generative AI and can be useful in helping government officials finish low risk but time consuming tasks faster like writing proposals or job descriptions.
Computer Vision
Computer vision trains computers to interpret and understand the visual world. Using digital images from cameras and videos and deep learning models, computer vision systems can accurately identify and classify objects, and then react to what they “see.”
The process involves collecting visual data, processing it, and then analyzing it to identify key patterns and features. This technology enables machines to perform tasks, such as recognizing faces, detecting objects in images, interpreting video feeds, and analyzing complex scenes. Computer Vision is widely used in various applications, including security systems, autonomous vehicles, quality inspection in manufacturing, medical image analysis, and interactive gaming. Its ability to process and understand visual information at a high speed and with great accuracy makes it an invaluable tool in many technological and industrial domains.
Natural Language Processing
Natural Language Processing or NLP allows computers to read text, hear speech, interpret it, measure sentiment, and determine which parts are important. NLP combines computational linguistics—rule-based modeling of human language—with statistical, machine learning, and deep learning models. The goal of NLP is to bridge the gap between human communication and computer understanding. This technology is used in a wide range of applications, including digital assistants, customer service chatbots, translation services, and tools for text analysis. It’s fundamental in making human-computer interactions more seamless and natural, especially in applications where understanding context and nuance in language is crucial.
Fuzzy Logic
Fuzzy logic can handle the concept of a partial truth, where the truth value may range between completely true and completely false. It’s particularly useful in systems that must make decisions based on ambiguous, imprecise, noisy, or missing information. This subset of AI enables more human-like reasoning and decision-making. A government application of fuzzy logic could be in adaptive traffic signal control. Fuzzy logic would allow traffic lights to adjust timings in real-time based on traffic conditions, pedestrian movement, and other factors like weather or special events. This could result in better traffic flow, less congestion, and could prioritize emergency vehicles, enhancing overall traffic efficiency and safety.
“AI in government isn’t just about buzzwords. It’s a transformative force with the power to revolutionize decision-making,” said UrbanLogiq CEO, Mark Masonsong.
“UrbanLogiq works to enable governments to harness this potential responsibly, breaking down data silos, ensuring data integrity, and fostering transparency. As we venture further into the realm of AI in governance, our holistic approach aims to unlock its full power for the benefit of the public.”
Example applications of AI in the public sector
Many governments are no strangers to AI. An example of that is fire professionals in the state of Minnesota. They’re using a Fire Analysis Solution that leverages AI to analyze various data sources. Analyzing historical fire incident reports, building characteristics, and census data helps identify areas and populations at higher risk of fires.
This predictive capability allows for better allocation of fire prevention resources, targeted public safety campaigns, and improved emergency response planning. The AI system’s ability to process and analyze large volumes of data quickly and accurately helps fire departments and emergency services be more proactive in their approach. Doing this potentially saves lives and reduces property damage by anticipating and mitigating risks before incidents occur.
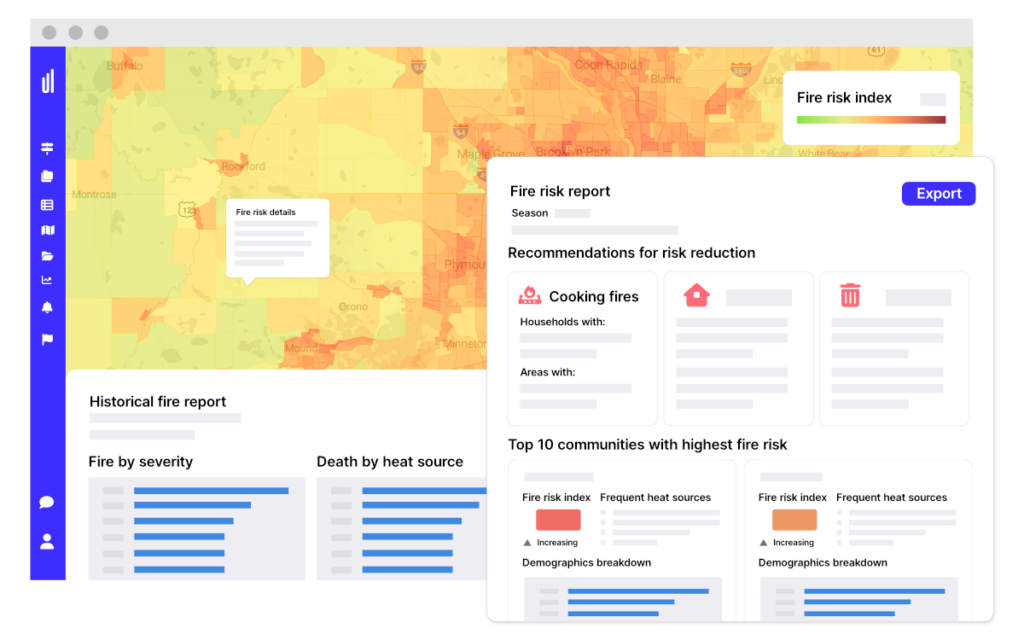
Image Source: UrbanLogiq
In Seattle, the Department of Transportation is exploring the use of Artificial Intelligene to enhance intersection safety. They’re conducting an 18-month pilot, using AI to analyze camera feeds at two intersections. The AI system works by distinguishing between different types of road users and understanding their movements, recognizing safety issues that may not be reported in police records. The technology focuses on detecting near-misses, which are more frequent than actual crashes. Providing a clear understanding of potential danger spots by generating a heat map to show where pedestrians are most at risk in an intersection, staff can proactively implement countermeasures to make the roads safer.
The diverse applications of AI in government sectors, ranging from traffic management to public safety and beyond, are just a glimpse into its expansive potential. However, each application brings with it a careful consideration of risk and reward. Ensuring that AI deployment is aligned with public interest, ethical standards, and compliance with regulatory frameworks is crucial. As we venture further into the realm of AI in governance, maintaining this balanced approach will be key to harnessing its power responsibly.
Garbage in is garbage out
A significant barrier to the adoption of more advanced AI forms is the quality of existing data. The cleanliness of government data is a critical factor in the effective use of Artificial Intelligence, underlining the principle of ‘garbage in, garbage out’. For AI systems to deliver accurate and reliable results, the input data needs to be high quality, well-organized, and free from errors. This is particularly challenging in the government sector, where data silos pose a significant hurdle. These silos, resulting from segregated data management across different departments or agencies, hinder the accessibility and consolidation of data. Without a holistic and integrated approach to data management, AI systems may struggle to access the comprehensive and coherent datasets they need to yield good results.
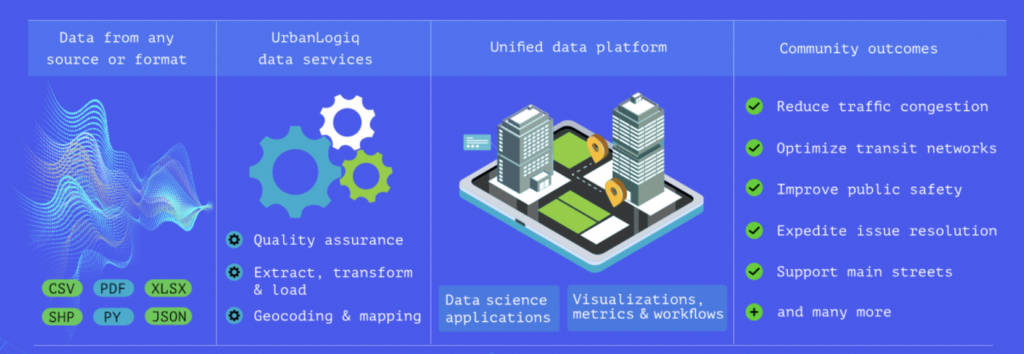
Image Source: UrbanLogiq
Preparing for AI Implementation
Implementing AI in government is not without its challenges. Ensuring data cleanliness and overcoming the barriers posed by data silos are essential steps for governments to fully realize the benefits of AI in their operations. Before diving into Artificial Intelligence, governments need to consider several factors:
Data Hygiene: Ensuring data quality is paramount. AI, especially deep learning, is only as good as the data it processes.
Governance Risk Model: Agencies need to develop a model to assess and manage the risks associated with AI deployment. Especially to determine which types of AI are appropriate for different use cases.
Data Compliance: Ethical implications, particularly around data privacy and the potential for bias, are crucial.
Data Security: Safeguards the integrity of the data against tampering or corruption is important to ensure that AI-driven results are reliable for informed decision-making.
How UrbanLogiq helps
UrbanLogiq offers a full-stack solution that enables government agencies to responsibly implement AI. UrbanLogiq’s platform facilitates the breaking down of data silos and ensures the integrity, safety, and compliance of data. By providing an end-to-end data platform, UrbanLogiq ensures the seamless integration of various data sets, which is crucial for leveraging the full potential of data through artificial intelligence. Integration is key to addressing concerns around security, privacy, and robust data governance. With its comprehensive approach, UrbanLogiq empowers government agencies to make more informed decisions, enhancing the efficiency and transparency of government operations.
Our story: https://youtu.be/hmp-eRd8OUg